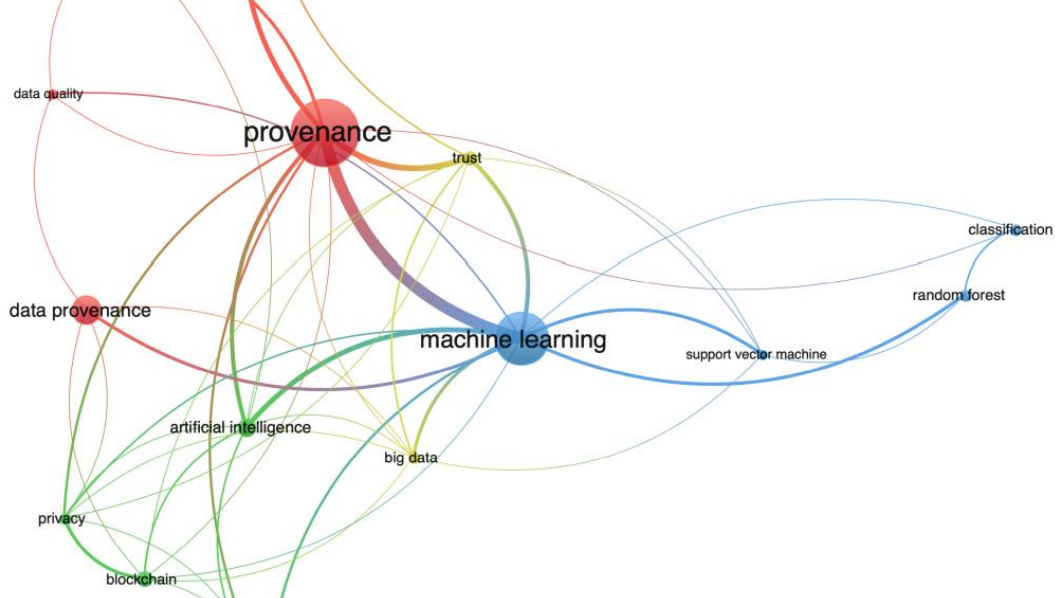
Doctoral Student Publishes Paper on Enabling Trustworthy AI
Computer Science doctoral student Amruta Kale had a paper published in Data Intelligence reviewing literature on Explainable Artificial Intelligence (XAI), identifying patterns in its recent developments, and offering a vision for future research. This paper from Kale and her collaborators at the University of Idaho and the University of Nevada, Reno is part of the TickBase project, led by Marshall (Xiaogang) Ma.
Artificial Intelligence (AI) and Machine Learning (ML) models have applications in numerous fields and are progressing rapidly. Results from these models can have a significant impact on human decision-making, but current models do not adequately explain how and why they get their findings. XAI holds substantial promise for improving trust and transparency in AI-based systems by explaining how complex models produce their outcomes.
Kale and her co-authors analyzed the relationship between XAI and provenanceāinformation about the data, algorithms, people, and activities involved in producing a result, which can be used to form assessments about its quality and reliability. They identified provenance documentation as a crucial part of building trust in AI-based systems.
The authors also presented a vision of expected future trends in this area. They anticipate researchers working to prevent biased and discriminatory results from AI and ML models, using traceable data, developing automated technologies for recording provenance information, and using a comprehensive approach to understand XAI from both its social and technical sides. They expect that through provenance documentation, more AI and ML models will become explainable and trustworthy.